🥯 Bread so lazy, it's always loafin' around
This edition covers OpenAI's video model, code snippets, CVs from YAML, fairness in AI, circular plots, and more ML insights.
Late to the Party 🎉 is about insights into real-world AI without the hype.
Hello internet,
I’m honestly enjoying the new vigour from the ounce of serotonin I get from the sun being out a bit.
In this issue, we have an OpenAIs video generation model, helpful code snippets, and CVs rendered from a YAML file. I talk about fairness in AI, circular plots, and a bunch of fun things I’m up to.
So, let’s dive right into some fascinating machine learning!
The Latest Fashion
OpenAI hypes up their Video Generation model Sora as a “world model.”
Some of Sebastian Raschka’s helpful code snippets for ML
I like this idea of rendering your CV from a YAML file!
Worried these links might be sponsored? Fret no more. They’re all organic, as per my ethics.
My Current Obsession
I accidentally hyperfocused and started collecting Python conference archives from all the way to the beginning, like PyCon US. It’s probably entirely useless for everyone, but it’s kinda of fun having those collected. Trying for others, but many Python conferences were announced on Listserves back in the day. Yes, PyCon is that old…
After a long while, I finally caved and had a cleaner come to my place again. If you’re neurodivergent like me and sometimes you just feel like you’re drowning in chores, I can highly recommend this. It’s less than $100, and my mind is so much more at ease right now that everything sparkles a bit and smells fresh around me. My anxiety is usually pretty high when I have this stranger in my house, but the end result is so worth it.
This week, it was announced that I will be a co-chair of the Working Group on Modeling for the ITU/WMO/UNEP Focus Group on AI for Natural Disaster Management, which is moving towards becoming a global initiative.
Next week, we’re holding the big machine learning training at ECMWF, so that takes up all my bandwidth at the moment. I still have some lectures to finalize. (And with finalize, I mean start, of course…)
Thing I Like
I got the new Pokemon Go Plus+ (what a terrible name), and I immediately had to modify it because the vibration was SO loud. But now I’m pretty happy with it. I get to play a bit while I’m on my way to work and while I’m on the go.
Hot off the Press
In Case You Missed It
Recently, my post on VSCode Extensions has been resurfacing. I should probably update it…
On Socials
People seem to be struggling with git!
My open PhD thesis is also quite popular!
Python Deadlines
I found Python fwdays, which closes in four days.
I’ve been doing a ton of work on the backend, updating Ruby and Jekyll and making the new Series feature robust. There are always those “cool things” that don’t see the spotlight but are necessary…
Machine Learning Insights
Last week, I asked, What methods do you recommend for ensuring fairness in AI algorithms, especially in high-stakes scenarios? and here’s the gist of it:
Ensuring fairness in AI algorithms, particularly in high-stakes scenarios such as healthcare, criminal justice, and finance, is not just a matter of preference but a critical necessity to prevent the potential harm of bias and discrimination. Here are several recommended methods to promote fairness:
Diverse Data Collection: The first step towards fairness is to ensure that the data used to train AI models is diverse and representative of all groups affected by the algorithm. Taking this step involves actively seeking out and including underrepresented groups in the data. Interestingly, under-sampled data is a primary source of bias in meteorological data.
Bias Detection and Mitigation Techniques: Implementing techniques to detect and mitigate bias in AI models is crucial. This can involve statistical methods to identify disparities in model performance across different groups and adjust the model or the data to reduce these disparities without pulling a Google Gemini and over-correcting.
Fairness Metrics Evaluation: Utilizing various fairness metrics can help assess whether an AI model is treating all groups fairly. Some common fairness metrics include equality of opportunity, demographic parity, and predictive parity. The choice of metric should align with the specific notion of fairness that is most relevant to the scenario.
Regular Auditing: Third-party audits of AI systems can help ensure that they continue to operate fairly over time. This involves technical evaluations of the models, their predictions, and broader impact assessments to understand how the AI system affects different groups. Possibly, this may even be mandatory, seeing as the EU AI Act just passed.
Explainability and Transparency: Using explainable AI makes it easier to understand decisions and identify and correct biases. This involves developing models that explain their decisions or using techniques to interpret complex models.
Ethical and Legal Frameworks: Developing and adhering to ethical guidelines and legal frameworks that mandate fairness in AI systems can provide a structured approach to addressing fairness. This includes both internal policies within organizations and external regulations like the EU AI Act.
Stakeholder Engagement: Engaging with and involving stakeholders, including those directly affected by the AI system, is not just a suggestion but a crucial part of the solution. Their insights into potential biases and fairness concerns are invaluable. This can also include collaboration with experts in ethics, sociology, and domain-specific areas.
Multi-disciplinary Approach: Tackling fairness in AI requires a multi-disciplinary approach, combining expertise from machine learning, social sciences, ethics, and domain-specific knowledge. This can help ensure that fairness measures are technically sound and socially relevant.
In high-stakes scenarios, where the consequences of unfair decisions can be particularly severe, these methods should be implemented with extra care and rigour. It’s crucial to understand that ensuring fairness is not a one-time task but an ongoing process, requiring continuous effort as models evolve and our understanding of fairness deepens. Your commitment to this process is vital.
Got this from a friend? Subscribe here!
Data Stories
Some visualizations thrive from being continuous.
pyCirclize makes this happen with different interfaces to create circular plots!
Of course, it’s not always the best choice.
But when it is…
It thrives!
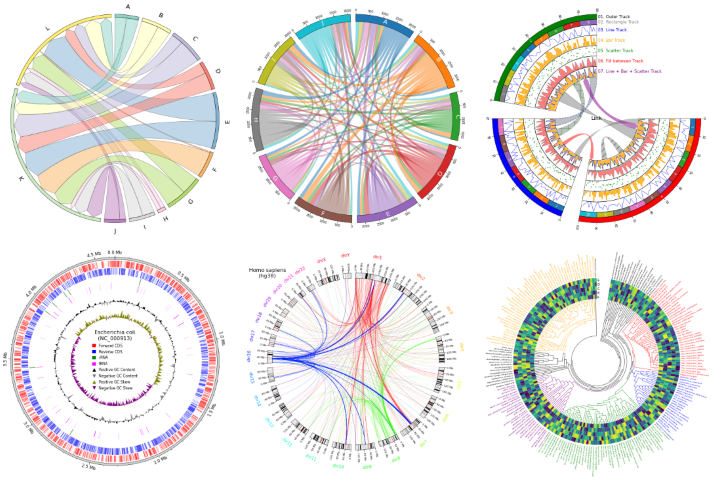
Source: PyCirclize
Question of the Week
How do you address the challenge of integrating diverse data types (like satellite imagery and ground sensor data) in ML models?
Post them on Mastodon and Tag me. I’d love to see what you come up with. Then, I can include them in the next issue!
Tidbits from the Web
I have been working too much the past week, so I’ll have to re-share some of my faves, as I barely have time for myself.
I always enjoy “Make Good Art” by Neil Gaiman.
Dropout’s Fantasy High is still my favourite evening entertainment currently.
Hunt: Showdown, my favourite game, just started a fun new event. Not for everyone, and 18+, so I won’t link today.
Jesper Dramsch is the creator of PythonDeadlin.es, ML.recipes, data-science-gui.de and the Latent Space Community.
I laid out my ethics including my stance on sponsorships, in case you're interested!